Global investment in AI systems has skyrocketed, surpassing a staggering $67.85 billion. This surge has ignited a burning question in the minds of businesses worldwide: how much does it cost to implement AI?
Unfortunately, there’s no one-size-fits-all answer to AI pricing. The development and maintenance of a bespoke intelligent system hinges on a multitude of variables, making AI project costs a unique estimation for each endeavor. This article aims to shed light on the primary cost drivers influencing AI projects. We’ll also equip you with ballpark figures to give you a general understanding of AI pricing.
Why Businesses are Embracing Artificial Intelligence
In today’s landscape, where COVID-19 continues to disrupt companies, waiting to adopt AI can be a costly decision. The need for automation is more critical than ever. Consequently, leading corporations are turning to intelligent systems to ensure business resilience in any situation. From streamlining in-house processes to revamping customer service, AI holds immense potential to automate workflows, leading to significant cost reductions.
Beyond this apparent advantage, smart applications unlock a treasure trove of benefits:
- Effective Data Management: AI empowers organizations to leverage the power of incoming data. For instance, AI technology can monitor equipment health, minimizing the risk of breakdowns or damage.
- Reduced Manual Input: By automating business processes, companies can expedite workflows. Compared to manual processing, AI performs complex tasks with greater efficiency and speed. Imagine automating tasks like sending emails, booking flights, and other mundane activities that drain valuable time.
- Predictive Analytics: AI-powered systems empower businesses to uncover hidden patterns and dependencies within their data. This translates to more effective utilization of Big Data and enhanced forecasting capabilities. Predictive analytics can also promote cross-selling opportunities, optimize inventory management, and improve daily operations.
- Security Maintenance: With data breaches exposing millions of records every year, robust security infrastructure remains a top concern. Fortunately, AI systems can assist companies in identifying fraudulent transactions and malicious activities. This facet of AI is particularly valuable for businesses developing fully autonomous systems or handling highly sensitive information.
This list merely scratches the surface of the advantages that automation brings to the table. It’s no wonder that companies prioritize AI strategies in a world dominated by COVID-19.
Challenges on the Road to AI Implementation
The cost of implementing AI is heavily influenced by the complexity of the automated system. Bringing an AI strategy to life, aligned with current business objectives, requires navigating some common challenges. Let’s delve into a few of them:
- Inaccurate Data: Most AI solutions thrive on high-quality data. They meticulously analyze every data point to generate new rules for business intelligence. Therefore, the AI implementation process hinges on securing the right dataset with complete and accurate information. Whether it’s labeled, unlabeled data, or search logs, companies need a well-stocked data repository for data scientists to build effective models. However, identifying the right data set can be a hurdle, especially for companies with vast amounts of unorganized information scattered across different systems. To address this challenge, many organizations seek guidance from AI professionals who can help them navigate the path to identifying the most suitable data for their AI projects.
- Data Security and Storage: While intelligent infrastructure can detect vulnerabilities, it can also introduce a new layer of susceptibility to cyberattacks and data leaks due to the massive volumes of data being analyzed. Additionally, the sheer amount of data can trigger storage concerns for businesses. Data-driven automation in business operations can introduce data security complications. Companies need to implement a robust data management environment that guarantees exceptional security against vulnerabilities while providing seamless access to data required for AI projects.
- AI Integration into Existing Systems: Established businesses are most likely to be the ones seeking AI adoption. However, integrating AI with existing business systems can be a struggle. This roadblock stems from the need to collect, clean, and unify data into a single, usable format. This spectrum of tasks encompasses various integration scenarios, such as data integration and improving overall usability. AI integration often makes up a significant portion of AI pricing since the vendor takes over a comprehensive suite of processes, from conception to deployment.
- Sophisticated Algorithms and Training: The effectiveness of your AI infrastructure rests heavily on the accuracy of its intelligent algorithms. However, training a model can be a time-consuming and data-intensive process. Furthermore, for your business intelligence to make real-world predictions, the model needs to be deployed in production. It’s also important to remember that AI-based algorithms necessitate continuous training and relevant data to maintain their accuracy levels. Therefore, ongoing maintenance of AI projects is another expense to consider when building your AI solution.
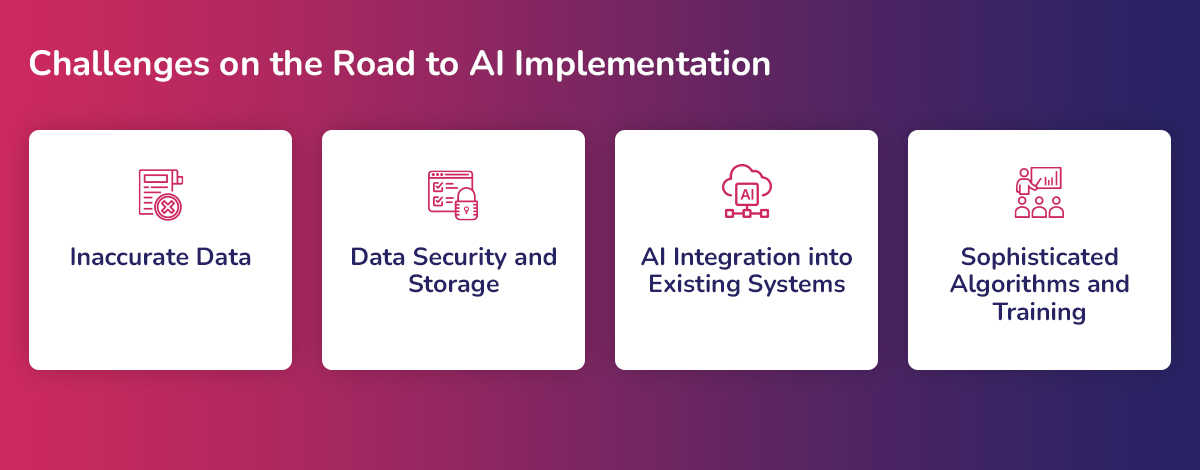
Unveiling the Cost Drivers of AI Projects
Just like any other tech project, AI pricing builds upon the fundamentals of basic estimation, with some unique adaptations. Here are four key factors that significantly impact AI project costs:
- Cooperation Model: There are various ways to approach AI development, with each model influencing the overall cost. Here are the common options:
- Outsourcing: Partnering with a third-party AI development company allows you to leverage their expertise and resources. However, outsourcing typically comes with a premium compared to in-house development.
- In-House Development: Building an AI team internally offers greater control over the project. However, this approach requires significant investment in recruiting and retaining skilled AI talent.
- Hybrid Model: This approach combines in-house development with outsourced elements. It allows you to leverage internal expertise while filling skill gaps with external resources.
- Project Management Triangle: The age-old project management triangle of time, cost, and scope applies heavily to AI projects as well.
- Time: The more complex your AI project, the more time it will take to develop and deploy. This translates to higher costs associated with developer hours and resource allocation.
- Cost: AI projects can range from tens of thousands of dollars to millions, depending on the factors discussed throughout this article.
- Scope: The features and functionalities you envision for your AI solution will significantly impact the overall cost. A more feature-rich solution will require more development time and resources, leading to higher costs.
- Core Features of Your AI Solution: The specific functionalities and capabilities you desire in your AI system will play a major role in determining the project’s price tag. Custom AI solutions, tailored to your unique needs, will generally be more expensive to develop compared to off-the-shelf solutions. While off-the-shelf solutions offer a quicker and potentially more affordable route, they may not perfectly align with your specific business requirements.
- Project Complexity: The intricacy of your AI project significantly influences the cost. Here’s a breakdown of some factors that contribute to project complexity:
- Data Complexity: The quality and structure of your data are crucial for building a reliable AI model. Complexities like missing data, unstructured data, and the need for extensive data cleaning will all contribute to higher costs.
- Algorithm Performance: The desired accuracy level of your AI algorithm is another cost driver. For instance, a facial recognition system requiring near-perfect accuracy will incur higher development costs compared to an application where a wider margin of error is acceptable.
AI Implementation: A Breakdown by Project Stage
As a complex technical solution, any intelligent system needs to be built incrementally. This means the implementation process unfolds across multiple stages, each consuming financial and human resources. Understanding how AI projects are priced at each development stage is crucial, especially if you’re considering hiring a third-party vendor to manage specific segments of the software creation process. The exact sequence and timeline of these stages can vary based on the scope and specific AI functionalities you want to integrate into your product.
1. Feasibility Study
Many AI projects require upfront research and development (R&D) activities to determine the most suitable delivery approach. This is particularly true for custom software development projects where there’s no straightforward path compared to off-the-shelf solutions. To kick off AI implementation, you might need to begin with a Discovery phase or a Proof of Concept (PoC).
In over 90% of cases, this initial phase typically requires a budget of $20,000 to $30,000. This upfront investment is crucial for validating your idea early on and gaining a clearer vision of the final product or solution. It also helps mitigate project failure risks and potentially save you money in the long run. For the development team, this stage is vital for identifying the most appropriate technical approach and the most cost-effective solution for your specific needs. The feasibility study also helps the vendor ensure you have the necessary data for the project’s success. AI projects can fail if the data is incomplete or difficult to obtain. Once the data is secured, the actual implementation often becomes the most streamlined part of the process. Here are some of the deliverables you can expect from this development stage:
- Problem Analysis: The development process begins with a thorough analysis of your business needs. The primary objective is to align project goals with the technical capabilities of your AI engineers. This stage also requires stakeholders to clearly articulate the business problem you’re trying to solve. The more specific you are, the more successful your AI implementation will be. Intelligent systems are typically designed to achieve attainable goals that enhance your company and support your software’s end-users. The cost of this stage can vary depending on the complexity of the problem. In some cases, a simpler solution that doesn’t involve machine learning might be a better fit. Additionally, some companies might just require professional guidance on acquiring the data necessary for the algorithm.
- In-Depth Business Analysis: A thorough business analysis is essential for engineers to grasp the project’s mission. With a clear understanding of the goals, developers can match your business requirements with the most optimal technical solutions. This analysis should result in detailed functional and non-functional requirements for the AI system, including accuracy rate, explainability, fairness, privacy, and response time.
2. Architecture Design
The duration of this stage hinges on the overall complexity of your software. Solution architecture bridges the gap between your business challenges and the technological solutions that will address them. Here’s a breakdown of what this stage entails:
- Identifying a suitable technological solution to address your specific business problem.
- Describe the structure, characteristics, behavior, and other components of the solution for stakeholders.
- Choosing appropriate integration patterns and procedures.
3. Software Development
This stage is the most labor-intensive part of the AI implementation process. Within AI development, it can be further divided into two phases:
- Front-End and Back-End Development: In this initial phase, developers construct the front-end user interface and back-end logic of the software, integrating APIs for the AI modules. Once the software undergoes successful testing, the development team can progress to building the actual intelligent system.
- AI Module Development: This phase primarily focuses on preparing data for the machine learning algorithm. Data-related tasks are often the most time-consuming aspect of AI development, contributing significantly to the overall cost. Here’s a closer look at what this phase entails:
- Data Preparation: ML engineers assess whether you have sufficient data for the project. If the data is tabular, it will be visualized for better comprehension. Data scientists will likely also need to cleanse the data to ensure all inputs are standardized and free of duplicates. If the data involves sensitive information, anonymization will be required.
- Data Collection (Optional): In the ideal scenario, a business will possess all the data required to train the model in-house. However, if your project lacks the necessary data or suffers from a lack of diversity, the team will need to collect additional data. Another hurdle can be raw data, which requires additional team members to markup the data for better training of the model.
- Baseline Model Creation: Once data scientists have secured all the necessary data, they will create a baseline model as a starting point. Baseline models are generally simple to set up and effective for achieving decent initial results. Additionally, they are cost-effective and allow for rapid experimentation. Baseline models also help engineers predict the accuracy of a more complex model. When the quality of the baseline model is satisfactory, the development team can progress to creating a more advanced machine learning model. Prototyping and Minimum Viable Products (MVPs) are also common practices to further validate the solution. Prototyping allows the team to test hypotheses about potential solutions by analyzing collected data. Viable hypotheses are then used to build the first version of the product, ready for launch. Once launched, the MVP will generate new data through user interactions. This user feedback is instrumental in refining the product’s quantitative and qualitative characteristics.
4. ML Model Training
This stage can take several weeks, depending on the complexity of the model. Here’s what occurs during this critical phase:
- ML Algorithm Selection: ML engineers meticulously select the most suitable machine learning algorithms to build the model.
- Model Training and Testing: The chosen algorithms are then trained using the training data and tested against a separate sample dataset. Engineers rigorously validate the final model against a test dataset within a pre-production environment.
5. Deployment
Finally, the solution reaches a point of equilibrium, ready for deployment. Vendors often recommend pilot deployment to a limited group of users to ensure a smooth rollout. The pilot deployment also helps verify compatibility with existing infrastructure and allows for user acceptance testing to eliminate any issues before a full-scale launch.
6. Maintenance
Software support is as crucial as software development. Therefore, factor in maintenance costs when building your AI budget. The development team will monitor the performance of the existing solution. Adaptation and retraining of AI components are frequent occurrences due to the influx of new data collected by the product. For solutions intended for public use, marketing your AI solution is another cost consideration.
How Much Does AI Cost in 2024 and Beyond?
Now that we’ve explored the intricacies of the AI development process, it’s evident that each stage requires financial resources. However, the most important takeaway is that you can initiate your project with an investigative stage costing around $20,000 to $30,000. This initial investment ensures the scalability of your project and provides a detailed estimation of the project’s timeline and overall cost.
The global landscape across nearly every industry is embracing AI to automate business processes and expand into new markets. This surge in demand has made AI software development a popular request among tech vendors. However, AI implementation is a complex endeavor that necessitates high-quality data, precise requirements, and effective algorithms to deliver real value. In most cases, affordability isn’t the primary concern. Instead, the focus should be on ensuring AI software development is cost-effective.
The total cost hinges on a multitude of factors, including the type of data your project requires, the intricacy of your AI system, and the desired accuracy level of your machine-learning algorithm. Overall, AI project costs can range anywhere from $20,000 to hundreds of thousands or even millions of dollars.
Remember, the investment you make today in digitally transforming your business through AI is an investment in your company’s long-term growth. It empowers your organization to respond swiftly to the ever-evolving challenges of today’s volatile, uncertain, complex, and ambiguous (VUCA) world.
Develop Your Next Big AI Project with QeDatalabs
Considering embarking on a complex AI project? At QeDatalabs, our skilled AI developers can provide consultations to assess the feasibility of your project and guide you through the entire development process. We understand the intricacies of AI development and can help you navigate the complexities involved in bringing your innovative ideas to life. Contact us today to discuss your AI project and unlock the potential of intelligent automation for your business!
